Flocking model
The flock model illustrates how flocking behavior can emerge when each bird follows three simple rules:
- maintain a minimum distance from other birds to avoid collision
- fly towards the average position of neighbors
- fly in the average direction of neighbors
Defining the core structures
We begin by calling the required packages and defining an agent type representing a bird.
using Agents
using Random, LinearAlgebra
@agent struct Bird(ContinuousAgent{2,Float64})
speed::Float64
cohere_factor::Float64
separation::Float64
separate_factor::Float64
match_factor::Float64
visual_distance::Float64
end
The fields id
and pos
, which are required for agents on ContinuousSpace
, are part of the struct. The field vel
, which is also added by using ContinuousAgent
is required for using move_agent!
in ContinuousSpace
with a time-stepping method. speed
defines how far the bird travels in the direction defined by vel
per step
. separation
defines the minimum distance a bird must maintain from its neighbors. visual_distance
refers to the distance a bird can see and defines a radius of neighboring birds. The contribution of each rule defined above receives an importance weight: cohere_factor
is the importance of maintaining the average position of neighbors, match_factor
is the importance of matching the average trajectory of neighboring birds, and separate_factor
is the importance of maintaining the minimum distance from neighboring birds.
The function initialize_model
generates birds and returns a model object using default values.
function initialize_model(;
n_birds = 100,
speed = 1.5,
cohere_factor = 0.1,
separation = 2.0,
separate_factor = 0.25,
match_factor = 0.04,
visual_distance = 5.0,
extent = (100, 100),
seed = 42,
)
space2d = ContinuousSpace(extent; spacing = visual_distance/1.5)
rng = Random.MersenneTwister(seed)
model = StandardABM(Bird, space2d; rng, agent_step!, scheduler = Schedulers.Randomly())
for _ in 1:n_birds
vel = rand(abmrng(model), SVector{2}) * 2 .- 1
add_agent!(
model,
vel,
speed,
cohere_factor,
separation,
separate_factor,
match_factor,
visual_distance,
)
end
return model
end
initialize_model (generic function with 1 method)
Defining the agent_step!
agent_step!
is the primary function called for each step and computes velocity according to the three rules defined above.
function agent_step!(bird, model)
# Obtain the ids of neighbors within the bird's visual distance
neighbor_ids = nearby_ids(bird, model, bird.visual_distance)
N = 0
match = separate = cohere = (0.0, 0.0)
# Calculate behaviour properties based on neighbors
for id in neighbor_ids
N += 1
neighbor = model[id].pos
heading = get_direction(bird.pos, neighbor, model)
# `cohere` computes the average position of neighboring birds
cohere = cohere .+ heading
if euclidean_distance(bird.pos, neighbor, model) < bird.separation
# `separate` repels the bird away from neighboring birds
separate = separate .- heading
end
# `match` computes the average trajectory of neighboring birds
match = match .+ model[id].vel
end
N = max(N, 1)
# Normalise results based on model input and neighbor count
cohere = cohere ./ N .* bird.cohere_factor
separate = separate ./ N .* bird.separate_factor
match = match ./ N .* bird.match_factor
# Compute velocity based on rules defined above
bird.vel = (bird.vel .+ cohere .+ separate .+ match) ./ 2
bird.vel = bird.vel ./ norm(bird.vel)
# Move bird according to new velocity and speed
move_agent!(bird, model, bird.speed)
end
model = initialize_model()
StandardABM with 100 agents of type Bird
agents container: Dict
space: periodic continuous space with [100.0, 100.0] extent and spacing=3.3333333333333335
scheduler: Agents.Schedulers.Randomly
Plotting the flock
using CairoMakie
The great thing about abmplot
is its flexibility. We can incorporate the direction of the birds when plotting them, by making the "marker" function agent_marker
create a Polygon
: a triangle with same orientation as the bird's velocity. It is as simple as defining the following function:
const bird_polygon = Makie.Polygon(Point2f[(-1, -1), (2, 0), (-1, 1)])
function bird_marker(b::Bird)
φ = atan(b.vel[2], b.vel[1]) #+ π/2 + π
rotate_polygon(bird_polygon, φ)
end
bird_marker (generic function with 1 method)
Where we have used the utility functions scale_polygon
and rotate_polygon
to act on a predefined polygon. translate_polygon
is also available. We now give bird_marker
to abmplot
, and notice how the agent_size
keyword is meaningless when using polygons as markers.
model = initialize_model()
figure, = abmplot(model; agent_marker = bird_marker)
figure
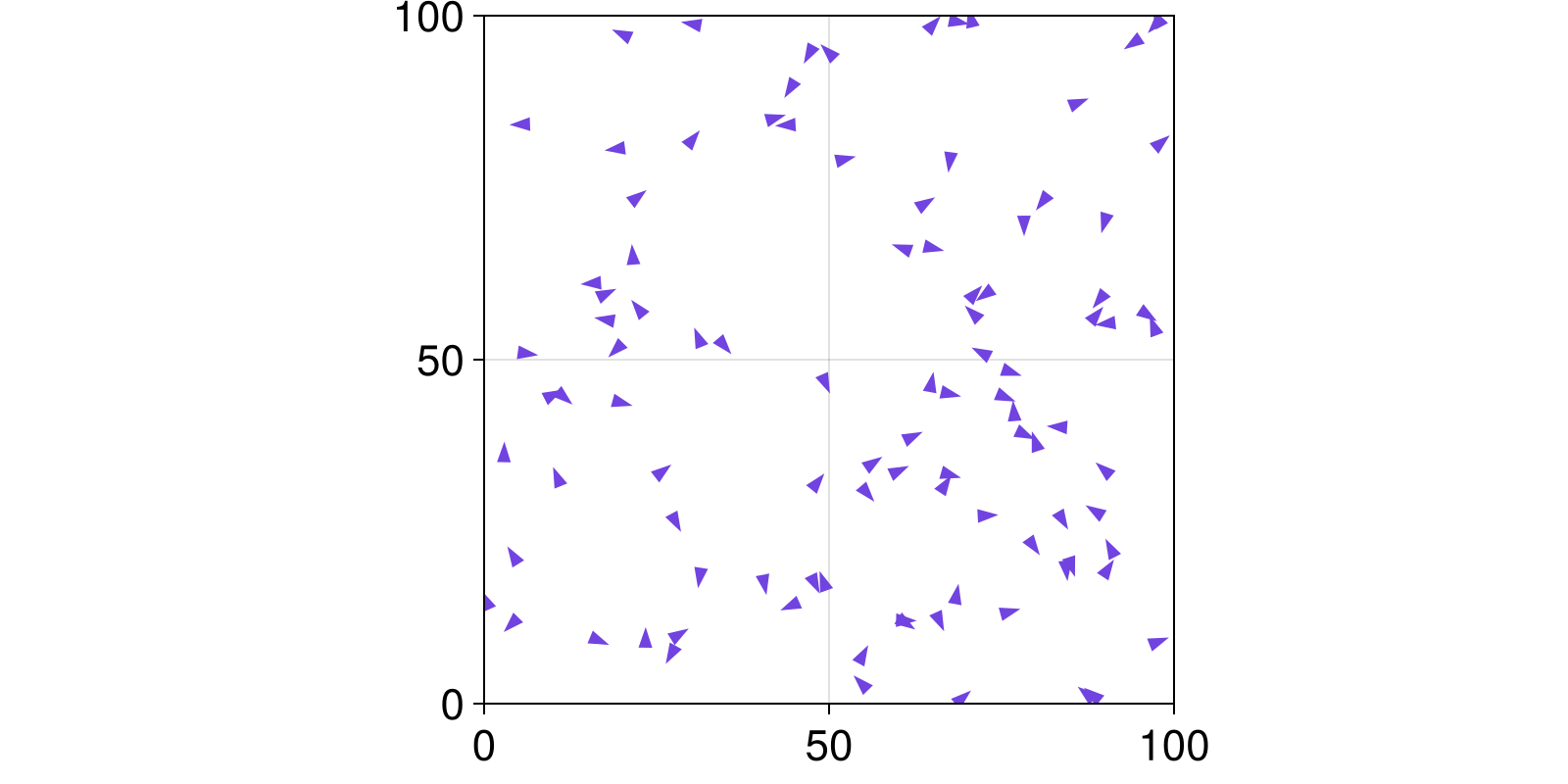
And let's also do a nice little video for it:
abmvideo(
"flocking.mp4", model;
agent_marker = bird_marker,
framerate = 20, frames = 150,
title = "Flocking"
)